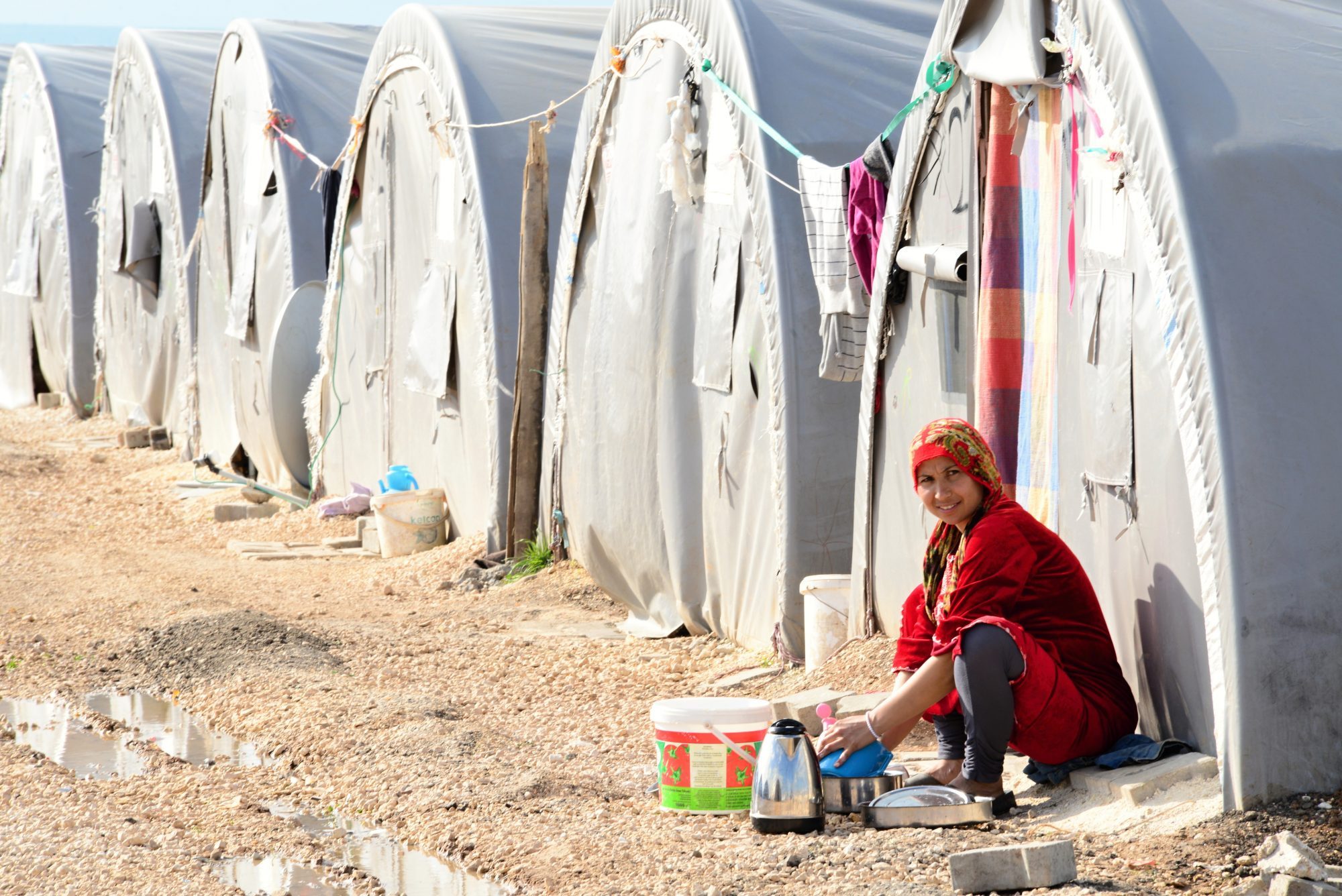
The global refugee crisis has ignited debate in nearly every country that has seen an influx of refugees and asylum seekers. In most countries, the conversation surrounding refugees has tended to focus on whether or not they should be allowed to enter. As policymakers and the press spar over quotas and security concerns, they have less often considered what happens to refugees after they arrive.
For many refugees, the road to integration is a rocky one. A refugee’s ability to acclimate or achieve economic success isn’t part of the selection criteria for admission—the few whom UNHCR selects for resettlement from refugee camps are those who can provide evidence of severe persecution and suffering. After experiencing the traumas of war and expulsion, refugees can find the challenges of acquiring a new language and finding basic employment to be insurmountable. These disadvantages all too often keep refugees on the margins of host countries’ economies and societies.
According to new research from the Immigration Policy Lab (IPL) at Stanford University and ETH Zurich, in conjunction with Dartmouth College, whether or not refugees land on their feet may depend on a critical factor that has been hiding in plain sight: where they are resettled within the host country. Their new city or town can act as either an obstacle or an on-ramp to successful integration, depending on the refugee’s profile. A good match can make a great deal of difference in helping refugees find work and put down roots. Aided by an IPL-designed algorithm, governments and resettlement agencies can now make the best matches possible—not just for a lucky few but for every resettled refugee.
The Power of Place
Why does the destination matter? After all, some refugees have the job and language skills to do well just about anywhere, and some locations boast strong labor markets and community-based organizations that would benefit any refugee. Yet the data reveal clear synergies between individuals’ characteristics and local conditions: some refugees’ strengths will be rewarded more in certain places than in others, while traits that might be liabilities in some places become less detrimental in others.
Currently, these synergies aren’t taken into account in any systematic way. In the United States, refugee arrivals tend to be sent to the location that has space to receive them at that moment in time. In other countries, such as Switzerland, asylum seekers are allocated randomly and proportionally across regions. Both countries have data on how refugees have fared economically in the past, but until now, the power of this information to improve outcomes for future refugee arrivals has not been realized.
IPL has developed a data-driven algorithm to optimize the process by which refugees are assigned to locations within a resettlement country. To picture the algorithm in action, imagine two refugees, both from the same country, of similar age, ethnic background, and skill levels. When resettled in different places, one thrives in finding work while the other struggles. The reasons their paths diverge are complex, but with an algorithm mining tens of thousands of historical cases, we don’t need to understand them fully in order to learn from them. The algorithm can detect systematic patterns, so the next time it receives a refugee who closely resembles these two, it will send him or her to the location where the earlier refugee prospered.
Take that basic idea and multiply it by a dozen individual characteristics and hundreds of potential resettlement locations, and you have a sense of what the algorithm can do if put in service of the thousands of refugees a country might receive in a given year. According to IPL affiliate Jeremy Ferwerda, assistant professor of government at Dartmouth College, “Algorithmic assignment holds the potential to simultaneously improve outcomes for refugees and the communities in which they are resettled.”
To build the algorithm, IPL’s researchers began with a modeling stage, using machine learning on historical data to calculate the likelihood that an individual refugee would find employment at each possible resettlement location within the host country, based on his or her demographic profile. Next, the team calculated the likelihood that at least one member of a refugee case or family would find a job at each location. From there, they matched each incoming refugee case to the location offering the highest probability of employment, taking into account real-world constraints such as the fixed number of available spots at each resettlement office.
To train the algorithm for use in the United States, the researchers used data on more than 30,000 refugees, aged 18–64, placed by a major resettlement agency from 2011-2016. Then they asked the algorithm to assign optimal locations for refugees who arrived at the end of 2016. The gains were striking: compared to the actual historical outcomes, the median refugee was more than twice as likely to find a job if placed by the algorithm. That’s an increase in employment probability from about 25 to 50 percent.
Refugees’ predicted employment rates rose across the board, including for those who were most and least likely to find work:
And nearly every resettlement location was better off: average employment rates at the vast majority of resettlement locations rose:
Tests also found that, had the algorithm been used, the average employment rate across all locations would have been 41 percent higher, rising from 34 to 48 percent.
When the tests were repeated in the context of Switzerland, the gains were even greater. With data from the Swiss State Secretariat for Migration, the researchers looked at asylum seekers who had been resettled across 26 regions between 1999 and 2013, and who had received subsidiary protection. After training the algorithm on the earlier data, the team tested it on asylum seekers who arrived in 2013. Their employment rate was 15 percent in actuality, but it would have been 26 percent had they been assigned to the algorithm-identified, optimal location—a 73 percent increase.
From Theory to Practice
The algorithm’s potential is all the more remarkable when compared with other possible interventions—like language instruction and job training—that, while essential, can be costly, logistically challenging, and difficult to scale. Algorithmic assignment is a rare policy reform that could deliver dramatic improvements at almost no cost. Notably, it would require little change to current bureaucratic procedures, says IPL data scientist Kirk Bansak: “Our goal was to develop a tool that not only worked well but was also practical from a real-world implementation standpoint. By improving an existing process using existing data, our algorithm avoids the financial and administrative hurdles that can often impede other policy innovations.”
This approach can also improve over time. The algorithm constantly mines updated data on refugee outcomes, so it will respond to changing conditions at each resettlement location, adjusting its assignments if certain refugees no longer fare well in places that were once their best match, or if better matches emerge. Finally, because it would propose top matches while offering the possibility of human override, the algorithm would complement, not replace, the expertise of government officials and resettlement officers responsible for delivering integration services.
Given the magnitude of the global refugee crisis, refugee-receiving countries need policy innovation if they are to continue to rise to the challenge. IPL’s data-driven approach to refugee resettlement presents a creative solution that can be implemented in any country that resettles refugees across domestic locations, offering a way to improve outcomes not only for refugees but also for the communities in which they live.
Watch: How the Algorithm Finds the Best Match
LOCATION
United States, Switzerland
RESEARCH QUESTION
How can we use machine learning to improve refugees’ outcomes?
TEAM
Kirk Bansak
Jeremy Ferwerda
Dartmouth College
Jens Hainmueller
Andrea Dillon
Dominik Hangartner
Duncan Lawrence
Jeremy Weinstein
RESEARCH DESIGN
Algorithmic assignment
KEY FINDING
Algorithmic assignment of refugees to resettlement locations could increase their employment probability by roughly 40-70 percent
FUNDER
Carnegie Corporation of New York