We develop a real-time scalable method to analyze strategic communication by political actors through their tweets. Using word embeddings and supervised machine learning methods, we classify legislators’ tweets according to whether or not they reference policy issue as well as what positions they promote. This allows us to measure the microdynamics of elite communication with a high level of temporal granularity in a manner that is scalable across diverse issue areas and legislatures. As a proof of concept, here we use this method to track the multi-year evolution of positions that members of Congress express on immigration and climate change. Validation with issue-specific vote scores suggests that our method performs with a satisfactory level of accuracy and enables us to identify legislators whose online rhetoric differs substantively from their voting behavior. We also display the immigration analysis on an automatically updated, publicly available interactive website, enabling researchers, journalists, and policymakers alike to explore legislators’ shifting rhetoric on immigration in real time.
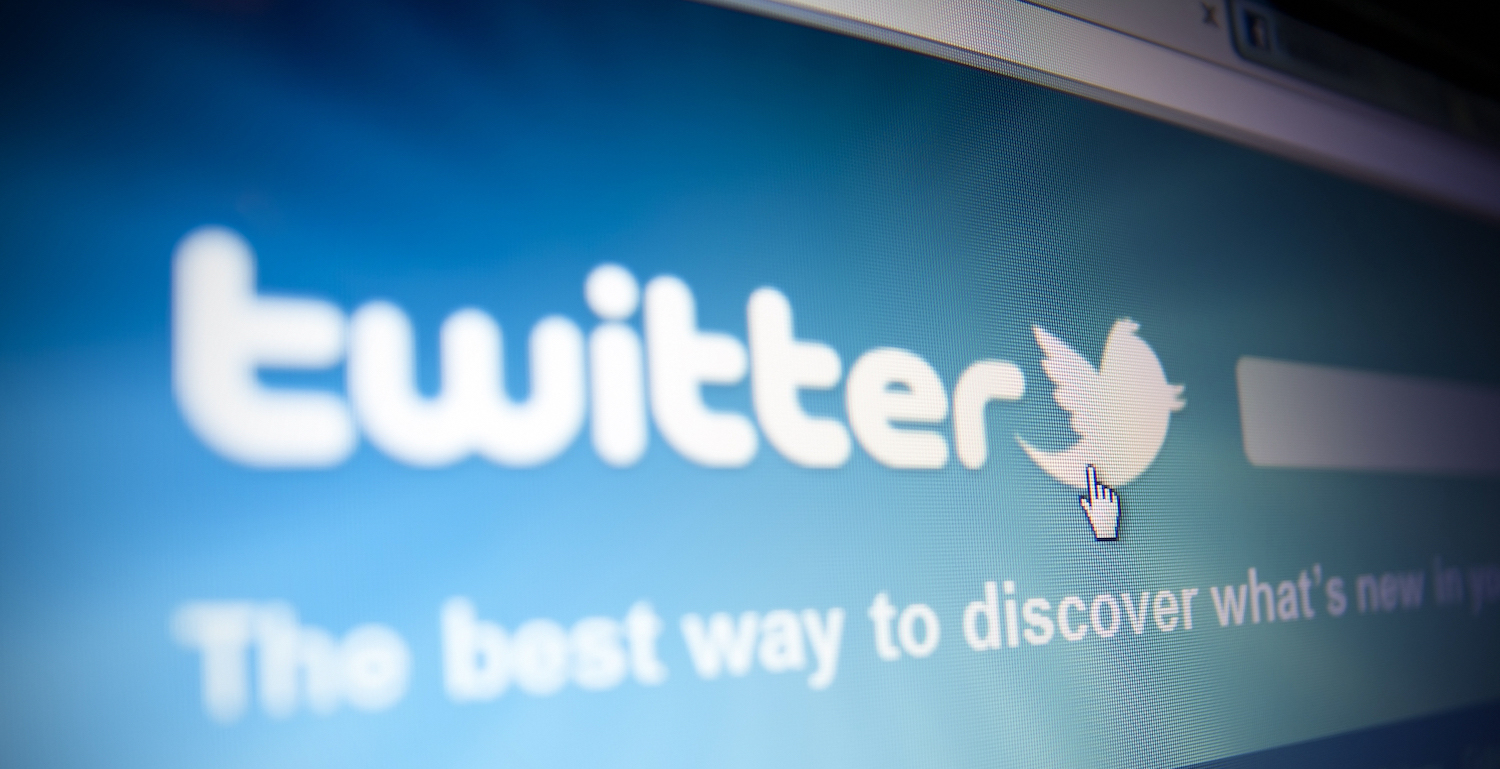
2022