A growing number of countries have established programs to attract immigrants who can contribute to their economy. Research suggests that immigrants’ initial arrival location plays a key role in shaping their economic success. Yet immigrants currently lack access to personalized information that would help them identify optimal destinations. Instead, they often rely on availability heuristics, which can lead to the selection of suboptimal landing locations, lower earnings, elevated outmigration rates, and concentration in the most well-known locations. To address this issue and counteract the effects of cognitive biases and limited information, we propose a data-driven decision helper that draws on behavioral insights, administrative data, and machine learning methods to inform immigrants’ location decisions. The decision helper provides personalized location recommendations that reflect immigrants’ preferences as well as data-driven predictions of the locations where they will maximize their earnings given their profile. We illustrate the potential impact of our approach using backtests conducted with administrative data that links the landing data of recent economic immigrants from Canada’s Express Entry system with their earnings, retrieved from tax records. Simulations across various scenarios suggest that providing location recommendations to incoming economic immigrants can increase their initial earnings and lead to a mild shift away from the most populous landing destinations. Our approach can be implemented within existing institutional structures at minimal cost, and it offers governments an opportunity to harness their administrative data to improve outcomes for economic immigrants.
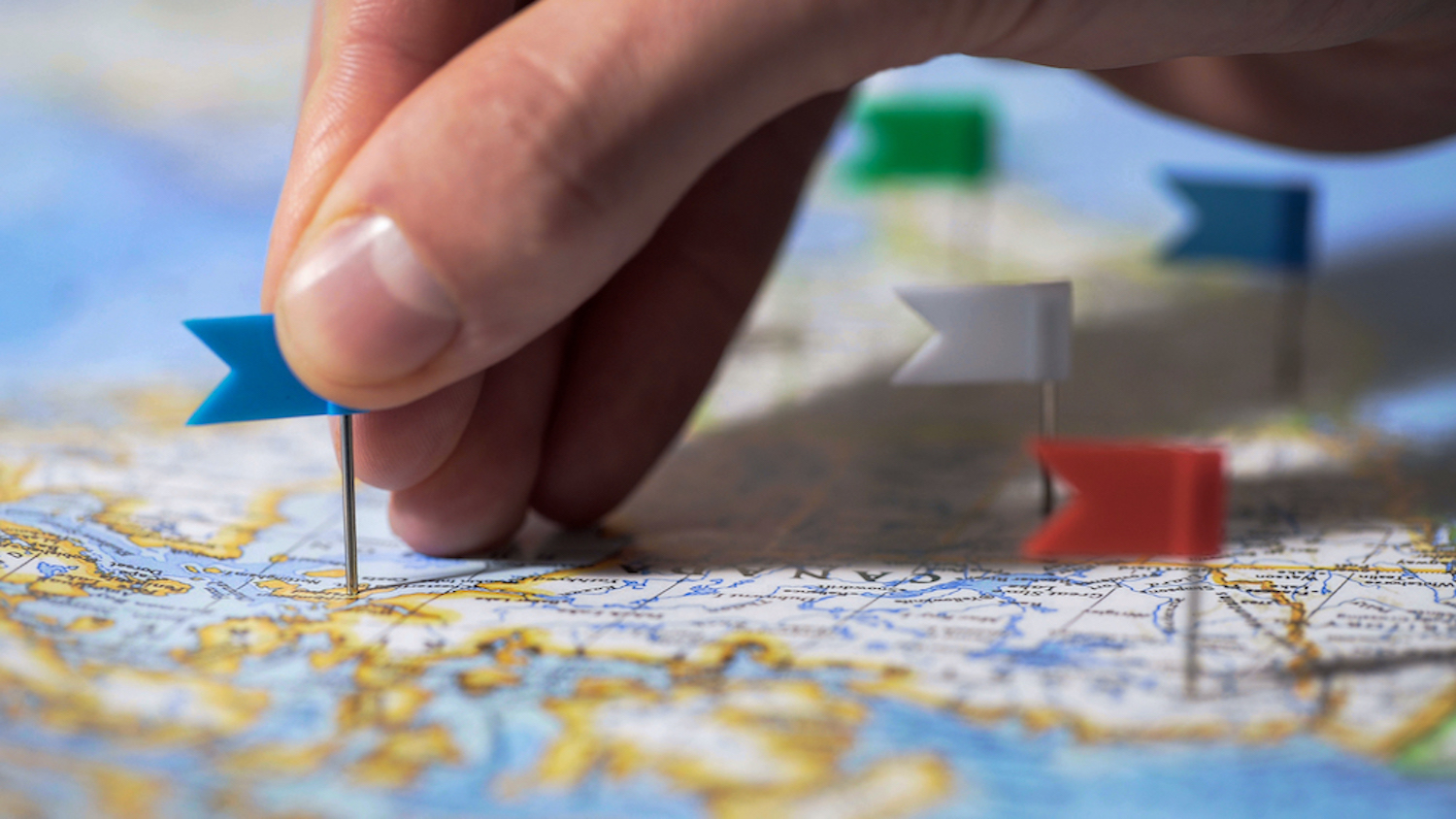
2020